FACULTY
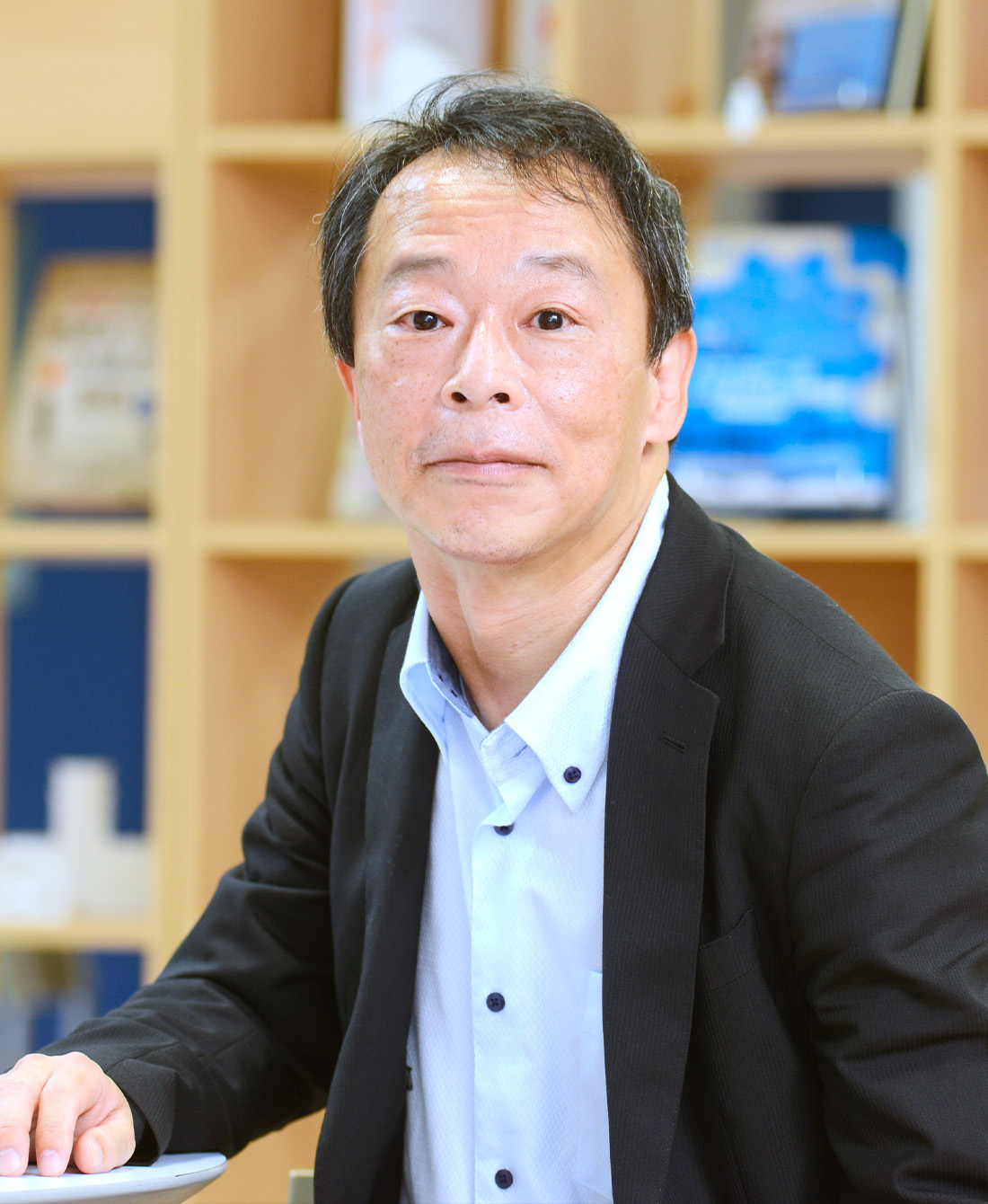
TOSHIAKI WATANABE
Graduate School of Social Data Science/Dean,Professor
Field of Study
Financial Econometrics
Macroeconometrics
Bayesian Econometrics
INTERVIEW
Unique Appeal of the Social Data Science Program
Today, a large amount of various data is available, and how to utilize such data is important in business and society. Data science is the development and application of methods to extract useful information from such data. However, in order to use data science to solve various problems in modern business and society, it is not enough just to learn data science technology. Knowledge of social sciences such as economics, business administration, marketing, law, and sociology is also required. The appeal of this faculty and graduate school is that you can systematically study social science in addition to data science technology. On top of that, in business innovation courses, social problem-solving courses, PBL exercises, seminars, etc., you can combine social science and data science to learn practical methods to solve business and social problems.・This is the charm of the graduate school.
Innovative education and research encouraged in the Social Data Science Program
In terms of education, I will be in charge of finance-related classes such as "Finance and Data Science" and "Financial Market Data Analysis" in the undergraduate course, and "Frequency Asset Price Data Analysis" in the master's course. In "Finance and Data Science", lectures will be given on the basic theory and empirical analysis of finance. In "Financial Market Data Analysis", we will give a lecture on how to analyze how asset prices fluctuate on a daily basis using time series analysis, and practice using actual stock price data. In "Frequency Asset Price Data Analysis", lectures and exercises will be given on the analysis of high-frequency asset price data during the day. In terms of research, we develop a time series model that expresses volatility fluctuations using high-frequency asset price data during the day and apply it to financial risk management. . Until now, we have mainly used the Bayesian estimation method as a metric method, but we would like to incorporate machine learning in the future.
CLASS
- (U)Financeand Data Science
- (U)Financial Market Data Analysis
- (U)Project-Based Learning Reserch C
- (G)Data Analysis of High Frequency Asset Pricing
- (G)Research Workshop Ⅰ・Ⅱ
RESEARCH
Research Website
My research is in the areas of econometric finance, macroeconometrics and Bayesian econometrics. In quantitative finance, I mainly study volatility, which expresses the variance or standard deviation of asset price change rates. Specifically, we use daily asset price data and intraday high-frequency data to develop a time-series model that expresses daily or intraday volatility fluctuations. It is applied to forecasting, derivation of option prices, and prediction of distribution tail risks such as value-at-risk and expected shortfall. In macroeconometrics, we are expanding the macro-theoretical model called the DSGE model and the macro-time series model called the VAR model. We also extend the Markov switching model and apply it to business cycles. In Bayesian econometrics, we are developing a Bayesian estimation method using the Markov chain Monte Carlo method (MCMC) to estimate the above model.
Keywords
- Bayesian statistics
- Business cycles
- Dynamic stochastic general equilibrium model
- Financial risk management
- High-frequency data
- Markov chain Monte Carlo
- Markov switching model
- Vector autoregressive model
- Volatility